Have you ever been invited to a friend’s new house and had them excitedly walk you through the improvements that they want to make as their family grows? When they get to the ideal location of their future master suite bathroom, you notice a problem - the plumbing goes nowhere near that future, perfect location in the house. It can be done, but it’s going to be costly and time consuming. I recently had an experience like that while assessing a Pega customer’s enquiry about where to apply Process AI.
Pega Process AI enables Pega’s customers to optimize the value of their workflows. Customers can apply machine learning and AI models to various decisions, such as what path to follow in a process, or to whom to assign work. It's not new technology, but rather a new application of the decision engine that has been driving customer engagement for some of the biggest companies on the planet for 10+ years.
My “Oh my, but there’s no plumbing here!” moment in a recent operational walk-through (see your friendly neighborhood Solution Consultant for a description of an operational walk-through) came during a deep dive of a pair of very successful workflows at a large customer, to assess them for optimization and for integration of Process AI. The workflows had multiple places where predictions could have a material, positive impact on the workflow KPIs, after some minor changes to how workflow data is captured and how workflow resolution is captured.
These workflows determined whether new business providers are legitimate businesses that will create non-fraudulent orders. The workflows guided the users through the processes of collecting information about new business providers, so that they could be added as a partner to process orders for the Pega customer on an on-going basis.
Hallmarks of a good Process AI workflow
At a high level, the customer’s workflows had all the hallmarks of great Process AI optimization candidates:
- Multiple outcomes that can be mapped to positive and negative results of the work
-
Multiple steps or stages that have varied or evolving activities
-
Stages and steps that can be skipped or abbreviated based on data captured in previous steps
-
Steps that can be optimized by skill -based or priority routing to different work groups
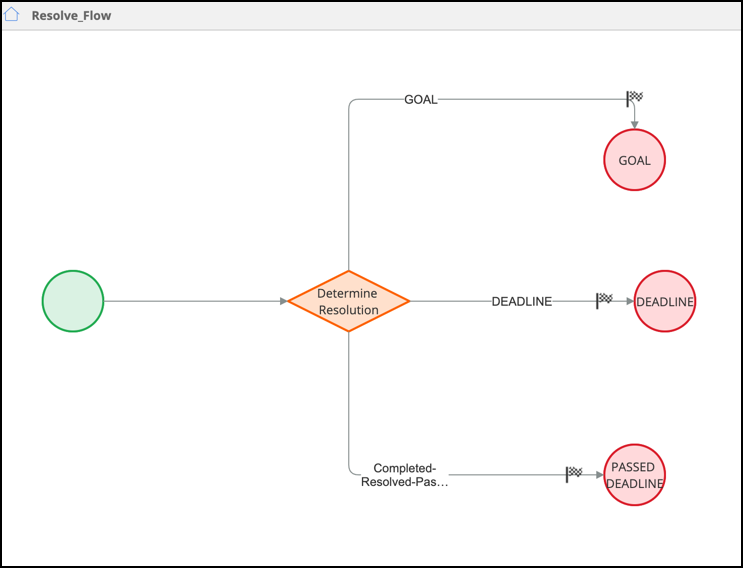
Workflow & Data Best Practices
To take advantage of those optimizations and prepare to apply Process AI, the customer needed to first do some planning and preparation - much like those friends in their new house will need to alter their designs to create their new bathroom. Some of the adjustments that we suggested to the customer to enable Pega Process AI to be more quickly applied included implementing:
-
Varied Outcomes: Workflow outcomes that are varied and can be associated with positive or negative results: Resolved-Completed-Goal, Resolved-Completed-PastDue, Resolve-Completed-Escalated
-
Common Values: Workflow properties that have a limited and specified range of values. For example, rather than just the URL text of an address, check the property that holds the URL and the property that records the conclusion of that check. For example, for an Address Check Result: Valid, Requires Investigation, Probable, Failed
-
Evolving Context: Capture workflow values in the steps in which the values are determined.
-
Consistent Value Ranges: Add integrations that systematically retrieve values wherever possible, to take human interpretation of the values out of play.
-
Systematic Decisions: Use workflow configuration to route the flow and assignment of work, and not manual processes, procedures, or communications that are external to the workflow. For example, email, sticky notes, or yelling across cubicles.
The images below show the difference between capturing data in a manual fashion and capturing the same data in a way that lends itself better to driving predictions:
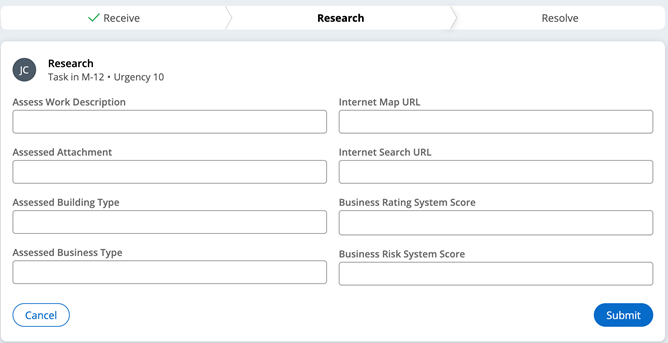
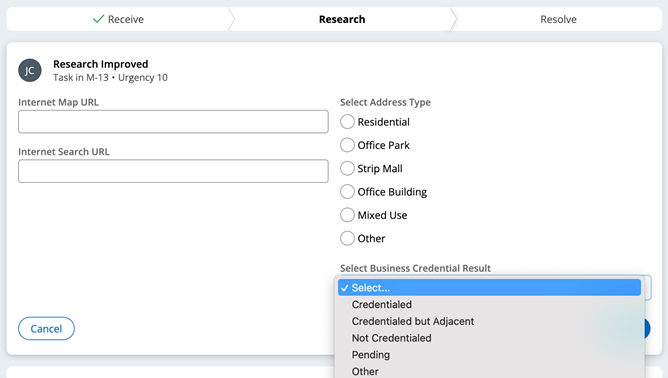
The 5 design guardrails described above are not only helpful in setting customers up to make use of Process AI optimizations, but are also best practices for any workflow. As an added benefit they can prevent you from making the “What do you mean I shouldn’t put a bathroom there?” face when you describe your plans to add Process AI to optimize your otherwise beautiful workflow.
Don't Forget
- JOIN THE CONVERSATION on Support Center
- FOLLOW @PegaDeveloper on Twitter